- August 3, 2022
- No Comment
- 99
Researchers Established a New System Using AI and ML to Control Digital Printing
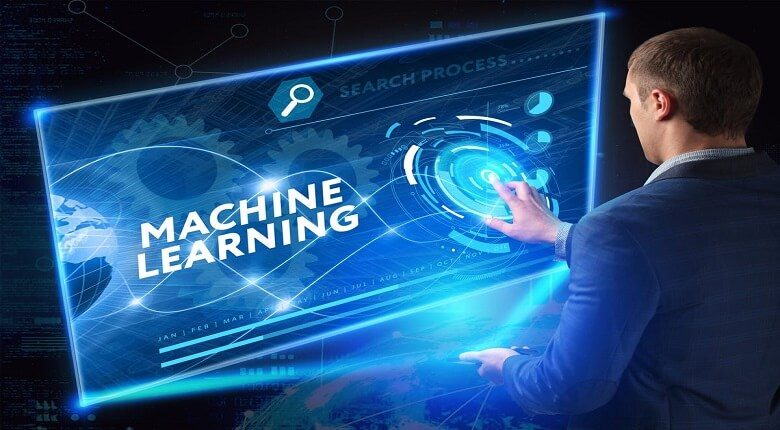
Tech giants and engineers are persistently developing new products with unique properties. Various firms use new inventions for 3D printing, while these products are considered complex and costly. However, professional operators often use manual trial-and-error to make thousands of prints. They decide the ideal magnitude to frequently print a new material efficiently.
These dimensions include printing speed and various other essential elements involved in the printing process. Now, MIT researchers have used artificial intelligence to reorganize the entire printing procedure. They established a Machine Learning system to utilize computer vision to monitor the manufacturing process. It can also correct errors and manage the material in real time.
This System Easily Integrates Novel Materials
The researchers used simulations to instruct a neural network to modify printing dimensions to reduce errors. It then implements these dimensions to controllers for a real 3D printer. These systems print objects more efficiently and perfectly compared to the other 3D printing controllers. More efficient work prevents the extremely expensive printing process for thousands of real objects to instruct the neural network.
The system allows engineers to easily integrate novel materials into their printing. It provides significant support to developing objects with special chemical or electrical properties. This approach helps operators in making adjustments to the printing process if environmental conditions or materials modify suddenly. A senior research author at MIT Wojciech Matusik issued a statement.
This Project Uses ML to Learn Complex Policies
Matusik said this project is obviously the first evidence of establishing a manufacturing system using ML to learn complex policies. More intelligent manufacturing machines can accommodate changing environments in real time at the workplace to enhance the perfection of the system. However, defining the perfect dimensions of a digital manufacturing process is considered the most expensive part of the process.
These dimensions are just ideal for one specific situation when a technician discovers a more efficient combination. However, machine learning or ML system is filled with challenges. The researchers should measure the happenings on the printer in real time. They have now established an advanced machine-vision system using 2 cameras designed at the nozzle of the 3D printer.
Neural Network Controller Manages Printing Process
Moreover, the vision system is considered a set of eyes monitoring the process in real time. The controller then processes images it collected from the vision system, adjusts the feed rate, and instructs the printer. But a well-trained neural network controller understands this manufacturing process that would need to make thousands of prints.
The researchers used a process of reinforcement learning to train their controllers. This model learns directly from trial-and-error with benefit. However, this model efficiently selects printing dimensions to create specific objects in a simulated environment. The model received benefits when the selected dimensions reduced errors during the printing process.
This Model Uses an Updated Control Policy
Meanwhile, the “error” means the model utilizes too much material for printing. It uses areas that should be left open or leaving spots in the printing process. The newly established model performs more simulated prints by updating its control policy to increase benefits with perfection. Keep in mind that the real world of printing is more critical than a simulation.
However, conditions traditionally change practically because of significant variations in various types of printing processes. The researchers have now established a numerical model that estimates noise and other factors from the 3D printer. They also added noise to this simulation model to get more realistic results. It enables transferring the control policy onto hardware specially trained in simulation.